What is the experimental method in marketing? As a Marketing professional — and even more so if you are a Growth Marketing professional — you already understand that the importance of Growth Experimentation Cycles cannot be overstated!
The Growth Experimentation framework describes where in the iterative process an experiment is and the stages of maturation that help growth marketers create clear expectations regarding outcomes (both in terms of planning and what to do once the results are in). Without Experimentation Cycles, experimentation and data analysis can easily get lost, and measurable outcomes can be hard to define.
Let’s take a look at all four stages and their impact.
Why are Growth Experimentation Cycles so important?
Growth Experimentation Cycles are a constant reminder of your marketing efforts, generating awareness around the objective and your confidence about the next steps. By being more objective about an experiment's stage and its results, you waste less time and fewer resources.
Growth Experimentation Cycles keep tasks on track, provide a guide for the next steps of experimenting, and allow a birds-eye view of the marketing process — Which experiments are we confident about? What brand new ideas are we testing?
Additionally, the Growth Experimentation Framework helps to define the ICE score — and as a result, help to prioritize the next stages of the process. This is a key trait of Agile Methodology, helping to move things forward and always be optimizing. This is the very foundation of growth as it relates to marketing.
The Impact of Defining Your Growth Experimentation Cycles
Experimentation Cycles help to define the ICE Score which helps to drive the next steps of every stage of a marketing process. Cycles provide us in-depth insight on:
- Confidence — How confident are we about this experiment's intended impact on our North Star?
- Timing — How long will we let this experiment run before defining the next steps? Resources — From where will we need to pull resources to help set up the next stages of an experiment?
- Knowledge — Have any questions been answered yet that help us define what these next stages look like? What new questions are we seeking to answer in our next steps?
The Stages of Growth Experimentation Cycles
Growth Experimentation Cycles are sorted into 4 main stages: Benchmark, Optimization, Validation, and Standardization.
Benchmark
A benchmark experiment is a first-time experiment with little to no supporting data. This is a net new experiment.
The confidence rating for a benchmark experiment is the lowest of all stages since there is no pre-existing data about this experiment. The timing in all experiment stages varies depending on the experiment type. However, because it is a new experiment, benchmark experiments should be checked on much more frequently than an experiment further along in the cycle. Resources in use for a benchmark experiment are the heaviest of all stages because everything is being built from scratch at this time. Optimization and knowledge will be essentially unknown aside from outside research that has been done.
Benchmark experiments are crucial for testing new ideas and first-time campaigns. Benchmark experiments are all about newness and context that will help us to define the following stages of our experiments.
Optimize
Growth Experiments in the Optimization cycle are narrower tests with benchmark data used as the guide. With an Optimization experiment, the idea is to change a single component to influence performance.
The impact on the confidence rating for an optimization experiment is the use of historical data from benchmark experiments. An optimization stage likely means that elements worth further testing have been defined in the benchmark stage of the experiment cycle and increase our confidence in the experiment. Timing, as with any cycle stage, is highly dependent on the type of experiment, though it may be shorter in this stage of the experiment. At this optimization stage, a 14-day window to analysis is typical. In contrast, resources for optimization experiments should be significantly reduced compared to benchmark experiments since you’re tweaking something existing vs creating something new.
At the optimization stage, knowledge is also heavily impacted. Optimization experiments start with stronger assumptions than benchmarks because they are being launched based off of a history of success. That also tends to lead to MORE questions being asked than answered though, because at this stage we're trying to learn WHY something worked — not just that it did — so it can be scaled and replicated for the correct reasons.
It is important to use this stage to define the WHY to eliminate time wasted, resources used, and improve future marketing strategy.
Validate
Validation experiments are tests of knowledge. In a validation experiment, we are testing elements of an experiment related to messaging, templates, and concepts as a whole. In other words, it allows us to test our winning concepts in additional environments: "We learned this thing, and we're going to prove that it works!”
Confidence levels for a validation experiment are quite high since we have already seen a win from these experiments and begun perfecting their formula in the optimization stage. Timing still varies per experiment type but may be shorter in a validation stage. Resources also vary, but will most often be much less than in both a benchmark or optimization experiment. Finally, knowledge should be pretty high at this stage — you have learned a lot previously and this stage isn’t so much answering more questions but is answering the questions: Will this always work? In how many different applications can we expect similar results?
It is at this stage that we start to transition from experimenting to growth marketing using concepts that are clearly defined by data and a process that is easy to replicate in multiple environments.
Standardize
At this stage in our marketing strategy, we have determined that something works, we have validated that it can be applied to multiple scenarios, and now we make it a standard of practice.
This is the point of scaling that we have worked so hard to get to. Now we have the proven data that we can point to as evidence that this model works. In the standardization stage of an experiment, our confidence and knowledge are at their highest, our resources should be minimal, and our timing is at it’s lowest score.
Standardization is about propagating our validated wins everywhere.
Navigating Growth with Experimentation Insight
In the ever-evolving realm of growth marketing, where strategies are tested and tactics are constantly refined, Growth Experimentation Cycles emerge as a guiding light. Experimentation Cycles provide growth marketers with a roadmap, allowing you to move through the landscape of uncertainties with confidence and data to back decisions. Resources and time are not wasted because experimentation cycles help to close knowledge gaps and set up the next steps. Scaling becomes not only possible but simple, with all of the steps to scaling mapped out ahead.
From laying the foundation with Benchmark experiments to fine-tuning with Optimization, and finally proving and scaling with Validation and Standardization, each cycle lends a hand in providing a roadmap to Growth.
As growth marketers, the goal is not merely to execute but to iterate, learn, and apply insights.
While embracing Experimentation Cycles is a choice, at Orange Pegs we believe that it is a necessity for those who seek strategic, data-driven growth. If growth is something you’re seeking, sign up for our Experimental Marketing Certification Series today.
Learn more about the lesson on Experiment cycles HERE:
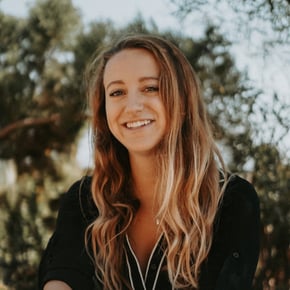